On this page: Series Overview • Key Focus Areas • Spring 2025 Events • Fall 2024 Events • Organizers • Attendance Information
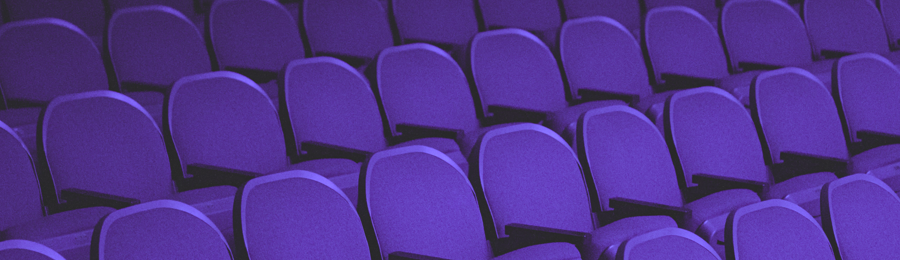
The CDS Seminar Series showcases the latest developments from all areas of data science, featuring local speakers, special interdisciplinary events, and the flagship CDS Colloquium with worldwide leaders in the field. It takes place every Friday from 2:00pm–4:00pm, with talks scheduled from 2:00pm–3:30pm, followed by a reception for attendees from 3:30pm–4:00pm.
Series Overview
Launched to highlight cutting-edge research, the CDS Seminar Series brings together experts from various disciplines within data science. The series aims to be accessible to the entire CDS community, fostering collaboration and sparking new ideas across different areas of the center. By featuring a diverse range of speakers and topics, the series provides a platform for knowledge exchange and interdisciplinary dialogue.
Key Focus Areas
The seminar covers a wide range of topics in data science, including but not limited to:
- Machine Learning
- Artificial Intelligence
- Natural Language Processing
- Computer Vision
- Statistical Modeling
- Data Visualization
- Big Data Analytics
- Neural Networks
- Reinforcement Learning
- Causal Inference
- Computational Biology
- Data Ethics and Fairness
- Deep Learning
- Bayesian Methods
This seminar series serves as a bridge between different parts of CDS, encouraging cross-pollination of ideas and fostering potential collaborations within the center.
Spring 2025 Events
- Date: Friday, April 25th
- Time: 2pm-3pm
- Location: 60 Fifth Avenue, Rm 150
- Speaker: Noga Zaslavsky
- Title: Information constrained emergent communication
- Abstract: While Large Language Models (LLMs) are transforming AI, they are also limited in important ways that are unlikely to be resolved with more data or compute resources. For example, LLMs require massive amounts of training data that does not exist for many languages, they are not grounded in the way humans perceive and act in the world, and they do not provide much insight on the origins of language and how languages evolve over time. In this talk, I propose a complementing approach. Specifically, I address the question: How can a human-like lexicon emerge in interactive neural agents, without any human supervision? To this end, I present a novel information-theoretic framework for emergent communication in artificial agents, which leverages recent empirical findings that human languages evolve under pressure to efficiently compress meanings into words via the Information Bottleneck (IB) principle. I show that this framework: (1) can give rise to human-like semantic systems in deep-learning agents, with an emergent signal-embedding space that resembles word embeddings, (2) yields better convergence rates and out-of-domain generalization compared to earlier emergent communication methods, and (3) allows us to bridge local context-sensitive pragmatic interactions and the emergence of a global non-contextualized lexicon. Taken together, this line of work advances our understanding of language evolution, both in humans and in machines, and more generally, it suggests that fundamental information-theoretic principles may underlie intelligent systems.
- Bio: Noga Zaslavsky is an Assistant Professor in the Psychology Department at NYU. Her research aims to understand language, learning, and reasoning from first principles, building on ideas and methods from machine learning and information theory. She is particularly interested in finding computational principles that explain how we use language to represent the environment; how this representation can be learned in humans and in artificial neural networks; how it interacts with other cognitive functions, such as perception, action, social reasoning, and decision making; and how it evolves over time and adapts to changing environments and social needs. She believes that such principles could advance our understanding of human and artificial cognition, as well as guide the development of artificial agents that can evolve on their own human-like communication systems without requiring huge amounts of human-generated training data.
- Date: Friday, April 18th
- Time: 2pm-3pm
- Location: 60 Fifth Avenue, Rm 150
- Speaker: Paul Röttger
- Title: Measuring Political Bias in Large Language Models
- Abstract: Large language models (LLMs) are helping millions of users to learn and write about a diversity of issues. In doing so, LLMs may expose users to new ideas and perspectives, or reinforce existing knowledge and user opinions. This creates concerns about political bias in LLMs, and how these biases might influence LLM users and society. In my talk, I will first discuss why measuring political biases in LLMs is difficult, and why most evidence so far should be approached with skepticism. Using the Political Compass Test as a case study, I will demonstrate critical issues of robustness and ecological validity when applying such tests to LLMs. Second, I will present our approach to building a more meaningful evaluation dataset called IssueBench, to measure biases in how LLMs write about political issues. I will describe the steps we took to make IssueBench realistic and robust. Then, I will outline our results from testing state-of-the-art LLMs with IssueBench, including clear evidence for issue bias, striking similarities in biases across models, and strong alignment with Democrat over Republican voter positions on a subset of issues.
- Bio: Paul is a postdoctoral researcher in the MilaNLP Lab at Bocconi University, working on evaluating and improving the alignment and safety of large language models (LLMs), as well as measuring their societal impacts. For his recent work in this area, he won Outstanding Paper at ACL and Best Paper at NeurIPS D&B. Before coming to Milan, Paul completed his PhD at the University of Oxford, where he worked on LLMs for hate speech detection. During his PhD, Paul also co-founded Rewire, a start-up building AI for content moderation, which was acquired by another large online safety company in 2023.
- Date: Friday, April 11th
- Time: 2pm-3pm
- Location: 60 Fifth Avenue, Rm 150
- Speaker: Nadav Brandes
- Title: Decoding the Genome with Large Language Models
- Abstract: Large language models are trained to predict the next token in a sequence. This seemingly simple task gives rise to incredibly powerful models with vast knowledge about the world. Like text, our genomes can also be modeled as sequences of tokens. A natural question to ask is: what would large language models trained on genomic sequences learn about our genome? Would they be able to absorb knowledge about the “meaning” of these sequences? The answer is a resounding yes. Models trained on protein sequences absorb critical knowledge about the structure and function of proteins, despite being trained on raw sequences. These models, it turns out, also learn which sequence variations are tolerated by evolution and are therefore likely benign, and which variants can cause disease. In fact, our work has shown that they are one of the most accurate tools we have for identifying pathogenic mutations, which has contributed to better diagnosis of genetic disorders. I’ll present these and other recent developments at the intersection of AI and genomics, and discuss some of the exciting opportunities they open to improve our understanding of the genome and diagnosis and treatment of disease.
- Bio: Nadav Brandes received his PhD in Computer Science from the Hebrew University of Jerusalem and completed his postdoctoral training at UCSF. In 2024, he joined NYU Grossman School of Medicine as an assistant professor in the Center for Human Genetics & Genomics and the Department of Biochemistry & Molecular Pharmacology. He is also affiliated with the Courant Institute of Mathematical Sciences and the Center for Data Science at NYU. His research focuses on harnessing the power of artificial intelligence to understand the human genome and address critical challenges in disease prediction and treatment.
- Date: Friday, April 4th
- Speaker: Michael Hu, Lily Zhang, & Falaah Arif Khan
- Speaker: Michael Hu (advised by Tal Linzen and Kyunghyun Cho)
- Title: Between Circuits and Chomsky: Pre-pretraining on Formal Languages Imparts Linguistic Biases.
- Abstract: Pre-pretraining language models on formal languages that capture natural language dependency structures effectively transfers to natural language. This approach reduces loss more efficiently than using the same amount of natural language, achieving equivalent loss with a 33% smaller token budget for a 1B-parameter model. Attention heads developed during formal language pre-pretraining remain essential for syntactic performance, providing mechanistic evidence of cross-task transfer.
- Speaker: Lily Zhang (advised by Rajesh Ranganath)
- Title: Preference learning made easy: everything should be understood through win rate
- Abstract: Preference learning, or the task of aligning generative models to preference comparison data, has yet to reach the conceptual maturity of classification, density estimation, etc. To close this gap, this work presents a framework to understand preference learning starting from the sampling distribution of pairwise preference data. First, we prove that the only evaluation of a generative model that respects both preferences and prevalences in the data distribution is a form of win rate, justifying win rate as the focal point to understand preference learning. We then analyze preference learning methods as win rate optimization (WRO) or non-WRO. We present novel instances of WRO beyond existing examples (RLHF, NLHF) and identify two key theoretical benefits of all such methods. We prove that common non-WRO methods like DPO and SFT on preferred samples lack these properties and suggest ways to mitigate such theoretical limitations. We also show that WRO underperforms in practice due optimization difficulties and that optimization success predicts performance better than choices which affect the objective’s solution. Our analysis highlights best practices for existing methods and provides recommendations for future research, guided by the principle that one should either align non-WRO methods more closely with WRO or improve the optimization of WRO objectives.
- Speaker: Falaah Arif Khan (advised by Emily Black and Sunoo Park)
- Title: Still More Shades of Null: Evaluating Missing Value Imputation Responsibly
- Abstract: Data missingness is a practical challenge of sustained interest to the scientific community. In this paper, we present Shades-of-Null, an evaluation suite for responsible missing value imputation. Our work is novel in two ways (i) we model realistic and socially-salient missingness scenarios that go beyond Rubin’s classic Missing Completely at Random (MCAR), Missing At Random (MAR) and Missing Not At Random (MNAR) settings, to include multi-mechanism missingness (when different missingness patterns co-exist in the data) and missingness shift (when the missingness mechanism changes between training and test) (ii) we evaluate imputers holistically, based on imputation quality and imputation fairness, as well as on the predictive performance, fairness and stability of the models that are trained and tested on the data post-imputation. We use Shades-of-Null to conduct a large-scale empirical study involving 29,736 experimental pipelines, and find that while there is no single best-performing imputation approach for all missingness types, interesting trade-offs arise between predictive performance, fairness and stability, based on the combination of missingness scenario, imputer choice, and the architecture of the predictive model. We make Shades-of-Null publicly available, to enable researchers to rigorously evaluate missing value imputation methods on a wide range of metrics in plausible and socially meaningful scenarios.
- Date: March 21, 2025
- Speaker(s): Ilia Sucholutsky & Shauli Ravfogel
- Title: Studying representational (mis)alignment: how and why? (Ilia Sucholutsky) / Representation-space interventions and their causal implications (Shauli Ravfogel)
- Overview:
- Ilia Sucholutsky: Do humans and machines represent the world in similar ways? Does it really matter if we do? I’ll share a simple method that anyone in the audience can immediately start using to study the representational alignment of their AI systems, even black-box ones, with humans or other models. We’ll then explore why we would want to do this by highlighting some examples of how representational alignment helps us learn more about both humans and machines and how it relates to key downstream properties of both machine learning and machine teaching.
- Shauli Ravfogel: I will introduce a line of work that focuses on identifying and manipulating the linear representation of specific concepts in language models. The family of techniques I will cover provides effective tools for various applications, including fairness and bias mitigation as well as causal interventions in the representation space. I will formulate the problem of linearly erasing information or steering the model towards one value or the concept, and derive closed form solutions. In the second half of the talk, I will address a key limitation of representation-space interventions—their opaqueness—and present two techniques for making these interventions interpretable by mapping them back to natural language: (1) learning a mapping from the latent space to text, or (2) deriving causally-correct counterfactual strings that correspond to a given intervention in the model.
- Date: March 14, 2025
- Speaker(s): Tom Griffiths
- Title: Using cognitive science to explore the symbolic limits of large language models
- Overview: Large language models are an impressive demonstration of how training a neural network on symbolic data can result in what looks like symbolic behavior. However, the limits of this symbolic behavior reveal some of the ways in which the underlying model and training regime can have unexpected consequences. I will highlight four such cases: ambiguous representations of numbers, influence of prior distributions on solutions to deterministic problems (“embers of autoregression”), paradoxical effects of chain-of-thought prompting, and implicit associations revealed through behavioral prompts. Each case makes use of specific tools from cognitive science — rational analysis, similarity judgments, and experimental methods designed to evaluate the impact of verbal thinking on behavior and reveal implicit biases.
- Bio: Tom Griffiths is the Henry R. Luce Professor of Information Technology, Consciousness and Culture in the Departments of Psychology and Computer Science at Princeton University, where he is also the Director of the new AI Lab. His research explores connections between human and machine learning, using ideas from statistics and artificial intelligence to understand how people solve the challenging computational problems they encounter in everyday life. He has made contributions to the development of Bayesian models of cognition, probabilistic machine learning, nonparametric Bayesian statistics, and models of cultural evolution, and his recent work has demonstrated how methods from cognitive science can shed light on modern AI systems. Tom completed his PhD in Psychology at Stanford University in 2005, and taught at Brown University and the University of California, Berkeley before moving to Princeton. He has received awards for his research from organizations ranging from the American Psychological Association to the National Academy of Sciences and is a co-author of the book Algorithms to Live By, introducing ideas from computer science and cognitive science to a general audience.
- Date: March 7, 2025
- Speaker(s): Brenden Lake (CDS)
- Title: Towards more human-like learning in machines: Bridging the data and generalization gaps
- Overview: There is an enormous data gap between how AI systems and children learn: The best LLMs now learn language from text with a word count in the trillions, whereas it would take a child roughly 100K years to reach those numbers through speech (Frank, 2023, “Bridging the data gap”). There is also a clear generalization gap: whereas machines struggle with systematic generalization, children can excel. For instance, once a child learns how to “skip,” they immediately know how to “skip twice” or “skip around the room with their hands up” due to their compositional skills. In this talk, I’ll describe two case studies in addressing these gaps.
- 1) The data gap: We train deep neural networks from scratch, not on large-scale data from the web, but through the eyes and ears of a single child. Using head-mounted video recordings from a child as training data (<200 hours of video slices over 26 months), we show how deep neural networks can perform challenging visual tasks, acquire many word-referent mappings, generalize to novel visual referents, and achieve multi-modal alignment. Our results demonstrate how today’s AI models are capable of learning key aspects of children’s early knowledge from realistic input.
- 2) The generalization gap: Can neural networks capture human-like systematic generalization? We address a 35-year-old debate catalyzed by Fodor and Pylyshyn’s classic article, which argued that standard neural networks are not viable models of the mind because they lack systematic compositionality — the algebraic ability to understand and produce novel combinations from known components. We’ll show how neural networks can achieve human-like systematic generalization when trained through meta-learning for compositionality (MLC), a new method for optimizing the compositional skills of neural networks through practice. With MLC, neural networks can match human performance and solve several machine learning benchmarks.
- Given these findings, we’ll discuss the paths forward for building machines that learn, generalize, and interact in more human-like ways based on more natural input, and for addressing classic debates in cognitive science through advances in AI.
- Date: February, 21, 2025
- Speaker(s): Emily Black (CDS)
- Title: Opportunities and Tensions for Fairness in AI & GenAI Systems
- Overview: AI and GenAI models are now ubiquitous in decision-making in high-stakes domains from healthcare to employment. Unfortunately, both AI and GenAI systems have displayed bias on the basis of race, gender, income, and other attributes. In certain domains– particularly credit, housing, and employment—this bias is often illegal. While AI governance frameworks are rapidly evolving, some of the strongest tools we have to combat this kind of discrimination in the United States are civil rights laws dating back to the 1960s. However, crucially, much of the academic work in the AI fairness space comes into tension with these laws—potentially making several state of the art techniques to mitigate and even test for bias unusable in high stakes domains such as credit, housing, and employment. In this talk, I’ll (1) illuminate these tensions between civil rights laws and AI debiasing methods as well as point out some technical insights into how to sidestep them, (2) demonstrate how these tensions have played out in practice in real-world high-stakes AI decision-making contexts such as fair lending, and (3) discuss some further, unique tensions to developing effective regulation to prevent AI harms for Generative AI systems.
- Date: February 14, 2025
- Speaker(s): Xi Chen (CDS)
- Title: Digital Privacy in Personalized Pricing and Trustworthy Machine Learning via Blockchain
- Overview: This talk has two parts. The first part is on digital privacy in personalized pricing. When involving personalized information, how to protect the privacy of such information becomes a critical issue in practice. In this talk, we consider a dynamic pricing problem with an unknown demand function of posted prices and personalized information. By leveraging the fundamental framework of differential privacy, we develop a privacy-preserving dynamic pricing policy, which tries to maximize the retailer revenue while avoiding information leakage of individual customers’ information and purchasing decisions. This is joint work with Prof. Yining Wang and Prof. David Simchi-Levi. The second part introduces the concept of using blockchain to create a decentralized computing market for any AI training/fine-tuning. We introduce the concept of incentive-security that incentivizes rational trainers to behave honestly for their best interest. We design a Proof-of-Learning mechanism with computational efficiency, a provable incentive-security guarantee, and controllable difficulty. Our research also proposes an environmentally friendly verification mechanism for blockchain systems, allowing existing proof-of-work computations to be used for AI services, thus achieving useful proof-of-work.
- Date: January 24, 2025
- Speaker(s): Eunsol Choi (CDS)
- Title: Equipping LLMs for Complex Knowledge Scenarios: Interaction and Retrieval
- Overview: Language models are increasingly used as an interface to gather information. Yet trusting the answers generated from LMs is risky, as they often contain incorrect or misleading information. Why is this happening? We identify two key issues: (1) ambiguous and underspecified user questions and (2) imperfect knowledge in LMs, especially for long tail or recent events. To address the first issue, we propose a system that can interact with users to clarify their intent before answering. By simulating their expected outcomes in the future turns, we reward LMs for generating clarifying questions and not just answering immediately. In the second part of the talk, I will discuss the state of retrieval augmentation, which is often lauded as the path to provide up-to-date, relevant knowledge to LMs. While their success is evident in scenarios where there exists a single gold document, incorporating information from a diverse set of documents remains challenging for both retrieval systems and LMs. Together, the talk highlights key research directions for building reliable LMs to answer information seeking questions.
Fall 2024 Events
- Date: November 8, 2024
- Speaker(s): Tim O’Donnell (McGill University)
- Title: Syntactic And Semantic Control Of Large Language Models Via Sequential Monte Carlo
- Overview: A wide range of LLM applications require generating text that conforms to syntactic or semantic constraints. Imposing such constraints nontrivially alters the distribution over sequences, usually making exact sampling intractable. In this work, building on the Language Model Probabilistic Programming framework of Lew et al. (2023), we develop an approach to approximate inference for controlled LLM generation based on sequential Monte Carlo (SMC). Our SMC framework allows us to flexibly incorporate domain- and problem-specific constraints at inference time, and efficiently reallocate computation in light of new information during the course of generation. We demonstrate that our approach improves downstream performance on four challenging domains—Python code generation for data science, text-to-SQL, goal inference, and molecule synthesis. We compare to a number of alternative and ablated approaches, showing that our accuracy improvements are driven by better approximation to the full Bayesian posterior.
- Date: November 1, 2024
- Speaker(s): Grace Lindsay and David Bau (of Northeastern University)
- Title: Finding Facts and Functions, and a Fabric (joint seminar with Minds, Brains, and Machines)
- Overview: In this talk we discuss recent work in interpreting and understanding the explicit structure of learned computations within large deep network models. We examine the localization of factual knowledge within transformer LMs, and discuss how these insights can be used to edit behavior of LLMs and multimodal diffusion models. Then we discuss recent findings on the structure of computations underlying in-context learning, and how these lead to insights about the representation and composition of functions within LLMs. Finally, time permitting, we discuss the technical challenges of doing interpretability research in a world where the most powerful models are only available via API, and we describe a National Deep Inference Fabric that will offer a transparent API standard that enables transparent scientific research on large-scale AI.
- Date: October 25, 2024
- Speaker(s): Eric Oermann, Krzysztof Geras, Narges Razavian
- Title: AI + Medicine
- Overview: A series of brief talks will be given by our Langone affiliates Dr. Eric Oermann, Dr. Krzysztof Geras, and Dr. Narges Razavian, followed by a lively panel discussion and Q&A moderated by Sumit Chopra.
- Date: October 4, 2024
- Speaker(s): Cédric Gerbelot-Barrillon and Jonathan Colner
- Title: High-dimensional optimization for multi-spiked tensor PCA (Cédric Gerbelot-Barrillon) and Leveraging foundation models to extract local administrative data (Jonathan Colner)
- Date: September 27, 2024
- Speaker(s): Byung-Doh Oh, Aahlad Puli, and Yuzhou Gu
- Titles: What can linguistic data tell us about the predictions of (large) language models? (Byung-Doh Oh), Explanations that reveal all through the definition of encoding (Aahlad Puli), Community detection in the hypergraph stochastic block model (Yuzhou Gu)
- Date: September 20, 2024
- Speaker(s): Nick Seaver, Kyunghyun Cho, Grace Lindsay (moderator: Leif Weatherby)
- Title: Oral History of Machine Learning – “Why We Call It “Attention”
- Overview: Attention has rapidly become an essential technique in AI. This conversation between Nick Seaver (Tufts, Anthropology), Kyunghyun Cho (NYU Center for Data Science), and Grace Lindsay (NYU Center for Data Science), looks back to a moment before attention became “all you need,” when attention mechanisms were imagined and introduced.
Organizers
The CDS Seminar Series is organized by Associate Professor of Mathematics and Data Science Jonathan Niles-Weed, Associate Professor of Mathematics and Data Science Carlos Fernandez-Granda, Associate Professor of Linguistics and Data Science Tal Linzen, and Assistant Professor of Mathematics and Data Science Yanjun Han.
Attendance Information
The CDS Seminar Series takes place every Friday from 2:00pm–4:00pm. Talks are scheduled from 2:00pm–3:30pm, followed by a reception for attendees from 3:30pm–4:00pm. All members of the CDS community are encouraged to attend these sessions featuring cutting-edge research and insights from leading experts in the field of data science.