On this page: Overview • Colloquium Series • Job Opportunities • Key NYU Faculty • Robert J. Glushko Undergraduate Thesis Prize
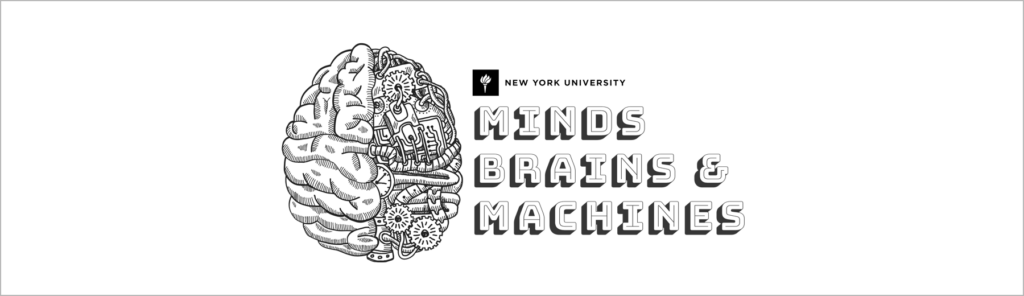
Overview
Understanding intelligence is one of the greatest scientific challenges, demanding an interdisciplinary approach that spans psychology, neural science, philosophy, linguistics, data science, and artificial intelligence (AI). At the core of this endeavor is computation—viewing intelligence as an advanced, adaptive computational process. However, the exact nature of the computation required for intelligence remains an open question. Despite striking recent progress in AI, current technologies provide nothing like the general-purpose, flexible intelligence inherent in humans.
A deeper dialogue between these fields is essential for transformative research, focused on two critical questions:
- How can machine intelligence enhance our understanding of natural (human and animal) intelligence?
- How can insights from natural intelligence guide the development of advanced machine intelligence that more fruitfully interacts with us?
NYU Minds, Brains, and Machines is a campus-wide initiative launched by FAS Dean Antonio Merlo. It aims to foster research and educational opportunities around these topics. The initiative started with a conference on human and machine intelligence and now prioritizes a hiring cluster that connects several academic units at NYU, including CDS, Psychology, the Center for Neural Science, and the Flatiron Institute.
Colloquium Series
The colloquium series showcases some of the most exciting work at the intersection of human and machine intelligence. Lectures will be held in person when possible.
Fall 2024 Events
David Bau (Northeastern University) and Grace Lindsay (CDS) – joint event with CDS Seminar Series
Date and Time: November 1, 2024, 2:00pm
Abstract: In this talk we discuss recent work in interpreting and understanding the explicit structure of learned computations within large deep network models. We examine the localization of factual knowledge within transformer LMs, and discuss how these insights can be used to edit behavior of LLMs and multimodal diffusion models. Then we discuss recent findings on the structure of computations underlying in-context learning, and how these lead to insights about the representation and composition of functions within LLMs. Finally, time permitting, we discuss the technical challenges of doing interpretability research in a world where the most powerful models are only available via API, and we describe a National Deep Inference Fabric that will offer a transparent API standard that enables transparent scientific research on large-scale AI.
Scientific Bio: David Bau is an assistant professor at Northeastern University Khoury school of Computer Sciences. He is a pioneer on deep network interpretability and model editing methods for large-scale AI such as large language models and image synthesis diffusion models. He is leading an effort to create a National Deep Inference Fabric.
Inbal Arnon (Hebrew University of Jerusalem)
Date and Time: September 23, 2024, 11:00am
Abstract: The learnability consequence and sources of Zipfian distributions: how cultural evolution can give rise to distributional properties of language. While the world’s languages differ in many respects, they share certain commonalities: these can provide crucial insight into our shared cognition and how it impacts language structure. In this talk, I explore the learnability consequences and sources of one of the most striking commonalities across languages: the way word frequencies are distributed. Across languages, words follow a Zipfian distribution, showing a power law relation between a word’s frequency and its rank. The source of this distribution has been heavily debated with ongoing controversy about what it can tell us about language. Here, I propose that such distributions confer a learnability advantage, leading to enhanced language learning in children, and the creation of a cognitive pressure to maintain similarly skewed distributions over time. In the first part of the talk, I will examine the learnability consequences of Zipfian distributions, focusing on their greater unigram predictability. In the second part, I explore the learnability sources of Zipfian distributions to ask whether learning biases can help explain why such distributions are so common in language. I will present joint work with Prof. Simon Kirby suggesting that this foundational distributional property of language can emerge through cultural transmission characterised by whole-to-part learning. I will end by discussing implications of these findings for large language models.
Scientific Bio: Prof. Arnon has a PhD in Linguistics and Cognitive Science (2011, Stanford University), and is currently a Full Professor of Psychology at the Hebrew University. Her research program, which lies on the interaction of Linguistics, Psychology, and Cognitive Science, focuses on understanding human’s unique ability to learn, use, and develop language, and more specifically, on understanding how children acquire language, how they differ from adults in doing so, and how learnability pressures shape the emergence and structure of human language. Prof. Arnon has worked extensively on first language acquisition, developing a novel framework for understanding why children are better language learners than adults, with applied implications for human and machine learning (The Starting Big Approach, see Arnon, 2021 for a review). In her current projects, she asks whether learning pressures and constraints can explain why languages look the way they do, and how linguistic structure emerged to begin with.
Past speakers include: Kelsey Allen (DeepMind), Ishita Dasgupta (DeepMind), SueYeon Chung (NYU), Steven Piantadosi (University of California Berkeley), Allison Gopnik (University of California Berkeley), Linda Smith (Indiana University-Bloomington), Michael C. Frank (Stanford University), and Kevin Ellis (Cornell university).
Job Opportunities
There are no current open faculty searches but stay tuned!
Key NYU Faculty
Here is a convenient listing of a few researchers working in this area along with a brief description of their research interests:
- Ned Block (Philosophy) – foundations of neuroscience and cognitive science, relationship between perception and cognition
- Sam Bowman (Linguistics/Center for Data Science) – natural language processing, deep learning
- David Chalmers (Philosophy) – philosophy of mind (especially consciousness), foundations of cognitive science, philosophy of language
- Kyunghyun Cho (Computer Science/Center for Data Science) – machine learning, natural language processing, deep learning (also research scientist at Facebook AI in NYC)
- SueYeon Chung (Center for Neural Science/Flatiron Institute – computational neuroscience, deep learning
- Ernest Davis (Computer Science) – commonsense understanding in human and artificial intelligence, physical reasoning
- Moira Dillon (Psychology) – cognitive development, spatial cognition, simulation vs. rule-based reasoning, human and artificial intelligence
- Rob Fergus (Computer Science) – machine learning and computer vision (also research scientist at Facebook AI in NYC)
- Todd Gureckis (Psychology) – active/self-directed learning, categorization, decision making, computational cognitive neuroscience, intersection of human/machine learning, crowdsourcing research
- Laura Gwilliams (Psychology) – cognitive neuroscience, language processing, auditory perception, models of speech comprehension
- Catherine Hartley (Psychology) – computational models of decision making and learning, developmental cognitive neuroscience
- David Heeger (Psychology/Center for Neural Science) – computational models of attention, perception, working memory, navigation, motor control
- Brenden Lake (Psychology/Center for Data Science) – computational cognitive modeling, human and machine learning, concept learning
- Grace Lindsay (Psychology/Center for Data Science)- computational models of sensory processing, human and machine learning, deep learning
- Tal Linzen (Linguistics/Center for Data Science) – computational models of human language acquisition and processing; evaluating and improving linguistic generalization in artificial intelligence systems
- Yann LeCun (Computer Science/Center for Data Science) – machine learning, computer vision, Director of AI research at Facebook in NYC
- Zhong-Lin Lu (Center for Neural Science/Psychology, Chief Scientist and Associate Provost for Sciences, NYU Shanghai) – computational brain models for perception and cognition, computational and psychophysics studies of perception, attention, perceptual learning, applications of hierarchical Bayesian models in adaptive testing, psychophysics, and brain networks
- Weiji Ma (Psychology/Center for Neural Science)- computational neuroscience, working memory, decision making, perception, higher cognition, fMRI
- Marcelo Mattar (Psychology)- reinforcement learning in biological and artificial systems, planning, memory, computational neuroscience, cognitive neuroscience
- Gary Marcus (Emeritus, Psychology) – cognitive scientist, AI entrepreneur
- Liina Pylkkänen (Linguistics/Psychology) – neural bases of language, particularly syntatic and semantic processing
- Bob Rehder (Psychology) – causal learning, categorization, reasoning
- Mengye Ren (Starting Fall 2022; Computer Science/Center for Data Science) – machine learning, computer vision, few-shot learning, brain & cognitively inspired learning
- Cristina Savin (Center for Neural Science/Center for Data Science) – using tools from machine learning to figure out how learning and memory happen at the level of neural circuits
- Eero Simoncelli (Center for Neural Science/Center for Data Science)- computer vision, computational approach to visual neuroscience
- Julian Togelius (CSE, Tandon School of Engineering) – artificial intelligence, evolutionary computation, games as AI testbeds
- Xiao-Jing Wang (Center for Neural Science/Simons Foundation/NYU Shanghai) – neurobiological principles of executive and cognitive functions
Robert J. Glushko Undergraduate Thesis Prize
The Glushko Prize for Outstanding Undergraduate Honors Thesis in Minds, Brains, and Machines is awarded annually to an NYU student who has conducted an Honors thesis in computational cognitive science or otherwise at the intersection of human and machine intelligence. Students in the Department of Psychology are eligible to apply if they complete an honors thesis or other independent thesis project.
The winning thesis will be the one demonstrating the greatest academic excellence and creativity using computational methods to inform aspects of intelligent behavior.
Two recipients will receive a monetary prize of $500 and will be recognized at their department’s Undergraduate Honors Ceremony in the Spring. In exceptional circumstances more than two prizes may be awarded in a single year. The Department will invite the winners to present their thesis in the following academic year (at a brown bag series, research lab meeting, etc.)
Students in Center for Data Science, Center for Neural Science, Linguistics, or Psychology who would like their thesis considered for this prize should have their faculty sponsor notify Todd Gureckis (todd.gureckis@nyu.edu) by April 1 of the spring semester (theses completed in the previous fall are still eligible for the spring).